Listen now
In the previous blog post, we explored how the current state of enterprise cybersecurity is broken due to highly manual workflows, increasing data silos, and an overwhelming volume of alerts, and we dug deeper into the transformative potential of foundation models in addressing these critical challenges.
We highlighted how AI-native approaches are poised to revolutionize key areas such as threat detection, incident response, and security training, while also noting the new attack vectors posed by malicious actors wielding similar technologies. Several emerging startups are at the forefront of this AI-driven transformation, reimagining traditional cyber workflows with innovative feature sets. Building on this foundation, we'll now take a deeper dive into how these new players are reshaping established cybersecurity categories and explore the essential strategies for success in winning over enterprise customers. By zooming in on specific examples and key success factors, we'll paint a clearer picture of the evolving cybersecurity landscape and the pivotal role of AI in shaping its future.
How are AI-native startups attacking the problem?
While enterprises (and investors) have been searching for the elusive "killer app" amidst the generative AI buzz, cybersecurity has emerged as the true game-changer, poised to revolutionize enterprise security paradigms and deliver tangible, mission-critical value. Let’s dive into some examples!
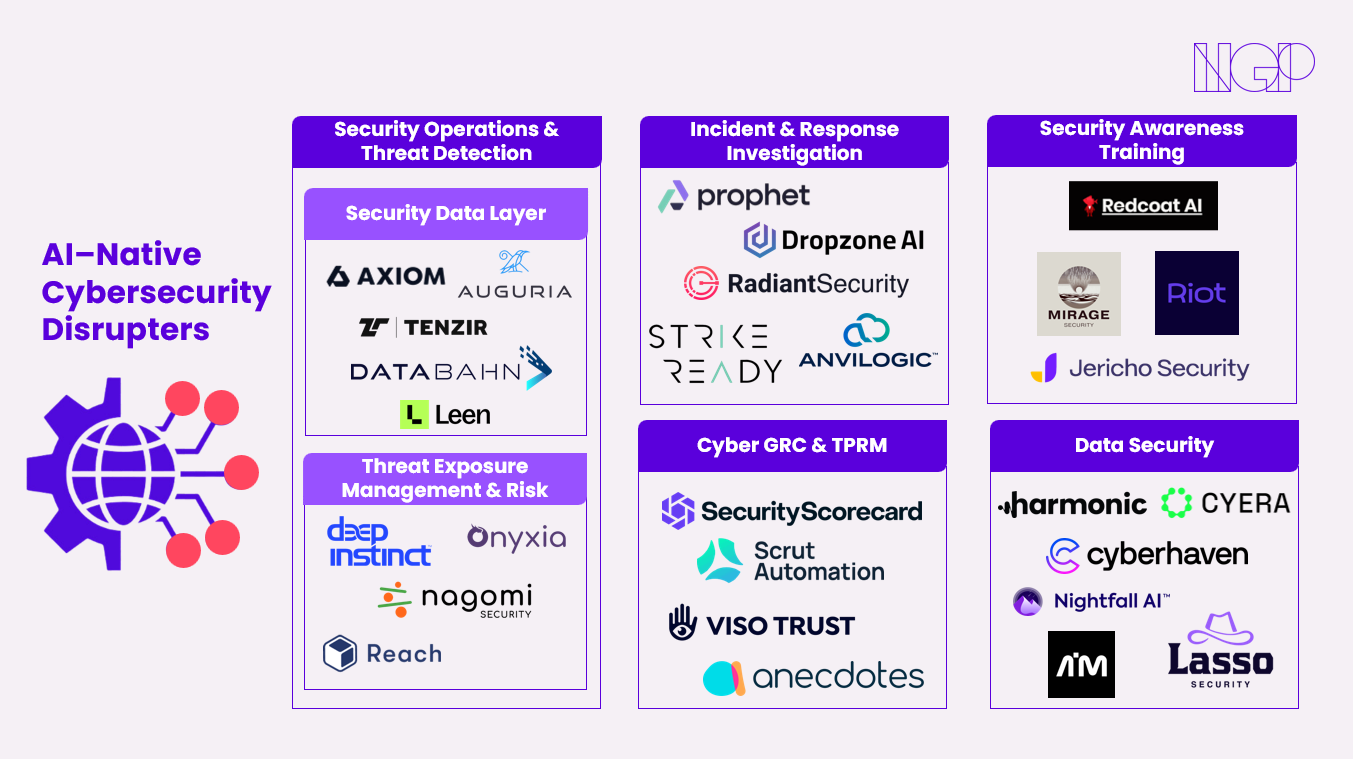
Security Operations & Threat Detection: Foundation models are transforming security operations by rapidly synthesizing log data across the siloed toolchain to autonomously identify vulnerabilities, generate detection rules, and prioritize threats according to business impact.
* Security Data Layer: Startups like Databahn, Tenzir, Axiom, and Auguria are modernizing system information & event management systems (SIEM) by building a unifying security data fabric or knowledge layer. These platforms focus on data pipelines—ingesting logs from existing security tools such as SIEM systems, data security platforms, identity providers, and threat intel feeds to elevate the alerts that have the highest level of business risk, while optimizing the volume and cost of data being sent to the SIEM. They have also been architected to leverage and support AI features with vector stores and fine-tuned security models available out-of-the-box, so customers can seamlessly integrate their own AI assets. This capability enables SOC teams to overcome alert fatigue, optimize ingestion costs, and allocate resources to the most critical security risks.
* Threat Exposure Management & Risk Quantification: Emerging players like Reach Security, Nagomi Security, and Onyxia help security teams understand risk posture, build proactive defenses, and optimize existing security tools. By analyzing historical incident data and threat intel feeds, LLMs can predict future attacks and simulate hypothetical scenarios, empowering organizations to proactively strengthen their cyber defenses and minimize the impact of breaches. This enables organizations to autonomously develop defensive actions and close existing detection gaps, which is crucial for staying ahead of evolving cyber threats.
Incident Response & Investigation: Automating incident response with LLMs drastically reduces the time needed to identify the cause of a breach, build incident response (IR) playbooks, and respond to alerts. Security operations teams struggle to manually piece together the relevant data across the toolchain to understand the criticality of the incident. New entrants like Prophet Security, Dropzone AI, and Radiant Security can synthesize complex alerts from disparate tools into a concise summary and response plan that is given to the SOC analyst in plain English—lowering mean time to respond (MTTR) while increasing alert capacity. These platforms also leverage reinforcement learning to continuously drive down false positives while autonomously optimizing remediation actions. This approach turbocharges the productivity of security operations teams and allows human analysts to shift their focus to the highest priority incidents.
Security Awareness & Training: LLMs can analyze an employee's job role, risk level, and security knowledge to help deliver individualized training materials that are relevant and insightful, while capturing real-time feedback. Large security awareness and training incumbent KnowBe4 announced a GenAI-enabled product that can proactively select security training modules or simulate phishing attacks that are hyper-personalized to the specific user. Mirage Security promises to build the world’s first AI autonomous multi-modal social engineering agent with new startups like Jericho Security and Riot Security taking a similar approach. The versatility of LLMs in adapting training content to different employee personas and experiences enhances overall security awareness, enabling organizations to mitigate the growing risks of highly personalized and more advanced social engineering campaigns.
Cyber GRC & TPRM: Emerging players like Scrut Automation and VISO Trust as well as our portfolio company SecurityScorecard are leveraging LLMs to automate compliance tasks such as risk assessments, compliance audit, and third-party risk questionnaires, freeing up resources for more critical operations. Additionally, LLMs can assist in mapping controls across the increasing number of data privacy and security regulations, ensuring organizations maintain regulatory compliance while optimizing operational efficiency.
Data Security: LLMs can classify sensitive data types (IP, financial models, engineering designs) with human-level accuracy. Instead of relying on manual review or superficial markers such as title, document type, or file size, innovators like Harmonic Security have built a network of small language models for classifying enterprise data types. They trained these models on a huge corpus of public data/documents to provide more granular visibility and controls for sensitive data (e.g. a user is exfiltrating an investment memo or sensitive design review). They also allow users to write data loss prevention (DLP) rules in plain English, which decreases the time and complexity for security teams to build out controls.
Keys to Winning the Enterprise
Emerging startups with AI-native approaches are poised to disrupt traditional workflows and create the next generation of cyber unicorns. That said, they will have to displace entrenched incumbents to carve out their unique positioning. Let's explore the four key strategies that will determine the success or failure of AI-native cybersecurity startups as they compete for enterprise dominance.
1. Disruption through complements: Each of the categories mentioned has large, formidable incumbents that are deeply entrenched in the enterprise. Additionally, cybersecurity platforms typically require significant user training and integration of critical systems, which makes displacing these solutions an extremely daunting task. Founders should focus on building complementary features that address blind spots within existing tools to reduce the friction of adopting a new platform.
2. Focus on ease of integration and user experience: Security teams are often understaffed and learning the capabilities of a new platform is challenging. To make matters worse, CISOs have battle scars from painful integration and deployment timelines. The next wave of AI-enabled solutions should focus on both ease of use and integration to maximize time-to-value without causing disruption.
3. Adopt composability to maximize performance: As open and close source models continue to improve, winning solutions will build composability where proprietary small language models are strung together with LLMs to solve more specialized cyber use cases. Many incumbents have significant technical debt that prevents them from easily integrating AI-enabled features or swapping models. The winners will be able to iterate quickly and use foundation model development as a catalyst for differentiation.
4. Prove ROI and beware of security tool sprawl: Security teams have limited budgets, and in the last 5 years, the rising complexity of the modern security stack has led to significant tool fatigue. Today more than ever, CISOs are hesitant to buy additional tools that don’t have a clear and tangible ROI. Founders should focus on building features that can drive immediate impact by increasing productivity through automation or decreasing the complexity of their existing tools.
__________________
That concludes our two-part series on how foundation models are disrupting cybersecurity! If you are building a company that is leveraging generative models to reimagine traditional cyber categories, I would love to connect via LinkedIn or email at eric@ngpcap.com. Always happy to chat if you have any feedback related to the post!