
Listen now
The exponential adoption of LLMs
Let’s face it. You don’t have to be a Gartner analyst to see that we are at the top of the hype cycle when it comes to generative AI. When OpenAI released ChatGPT in November 2022, the average consumer was able to get a first-hand look at how far we’ve come since Google Brain’s Transformer paper and, more importantly, how transformative LLMs (Large Language Models) can be to everyday work and life.
The adoption curve looks more like a SpaceX launch than even the exponential growth of the most viral apps of the century like Instagram or TikTok—reaching 1 million users in 5 days and 100 million users in less than 2 months. Where the internet made information accessible, the adoption of LLMs (Large Language Models) is democratizing synthesis. Users ranging from fifth-grade students to cybersecurity leaders are leveraging this technology to enhance their current capabilities.
As user adoption scaled, then came the wave of developers who began scrambling to find high-value use cases that could be automated or reimagined without spending hundreds of millions of dollars on model development and training, and instead using the library of APIs that were released by the foundation model providers (OpenAI, Anthropic, HuggingFace, to name a few of the most performant players).
According to Databricks' 2023 State of Data + AI Report, the number of companies using SaaS LLMs APIs has grown 1310% from November 2022 to the beginning of May 2023. No wonder VCs and the like started to shout from the rooftops, “the next iPhone moment is upon us,” which could very well be true.

As large language models scaled in size (both number of parameters and training tokens), their capabilities continued to increase, providing even more incentive for incumbents and new disruptors to build or augment entire businesses on top of these models.
Although fine-tuning APIs were released by some of the closed-source incumbents, there was still significant friction to widespread adoption: data security and privacy challenges, training costs, and uptime concerns given the models aren’t hosted on internal infrastructure. However, a fortunate 4chan leak of Meta’s LLaMA in early March led to a flood of innovation and experimentation in the open-source realm. The developer community demonstrated that bigger isn’t always better when deploying LLMs, and new fine-tuning techniques could significantly drive down training costs without affecting performance. Examples include Vicuna and Koala, two models that maintained performance thresholds near their Big Tech competitors with a small fraction of the training costs, $300 and $100 respectively.
Why is this important for the application layer? Well, these developments proved open-source models can perform just as well at use case specific tasks with palatable training costs for both large enterprises and new startups. This development suggested that the model layer will become commoditized (Google—We Have No Moat, And Neither Does OpenAI), shifting the focus to the application layer.
So, how can startups build defensibility in the application layer of generative AI? Let’s explore five strategies founders can take to fortify their position in the application layer and carve out a distinct competitive advantage.
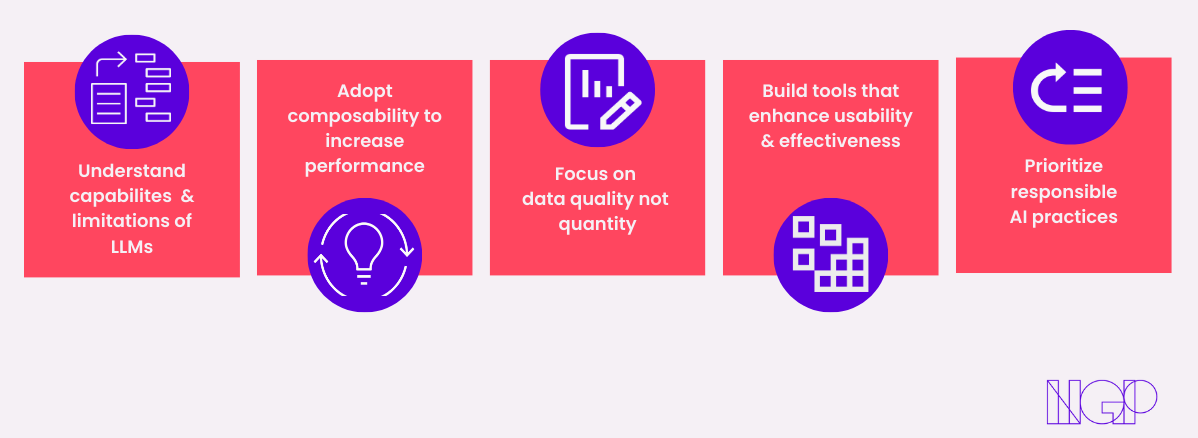
1) Understand capabilities & limitations of LLMs
Understanding the capabilities and limitations of LLMs is the first step. While these models can generate human-like text and are powerful tools for more general tasks like content creation and text summarization, they also have limitations, such as a lack of industry or use-case-specific context, the potential for hallucination, exuberant training, and usage costs, and sensitivity to input phrasing.
2) Adopt composability to increase performance
Given these characteristics, one strategy for developing long-term value and differentiation is to build composability where proprietary models are strung together with LLMs to solve more specialized, industry-specific use cases. This could involve fine-tuning both in-house and closed-source models for specific subtasks within a larger workflow. For example, NGP portfolio company Observe AI has been using BERT in parallel with in-house models to provide contact center agent coaching. Their success is predicated on the large corpus of relevant transcripts they have acquired over the last 5 years.
3) Focus on data quality, not quantity
Another strategy is to focus on the quality of data rather than the quantity. As LLMs become more commoditized, the value will shift towards the data used to train and fine-tune these models. Companies that can gather proprietary, high-quality data that is difficult for others to replicate will have a strong competitive advantage. This could involve investing in data collection and annotation efforts, developing partnerships to access proprietary data, or creating feedback loops to continuously improve the data over time. I would argue that Nuance Communications was bought by Microsoft in April 2021 for $19.7B because of the data moat the company had acquired.
4) Build tools that enhance usability & effectiveness
In addition to data, another key area of differentiation is the development of workflows and tools that enhance the usability and effectiveness of LLMs. This could involve creating user-friendly interfaces, developing tools to monitor and control the outputs of the models, or integrating the models into existing software and systems. The LLM then becomes one piece of the value chain within a more complex workflow with the output being an automated action. Defensibility will be created in the volume of integrations and ease of use. For example, although ChatGPT in itself is powerful, the development of plugins exponentially amplified its power by enabling the platform to work with existing applications to provide end-to-end functionality. Ultimately, companies that can make LLMs more accessible to end users will have a significant advantage.
5) Prioritize responsible AI practices
Finally, given the ethical and societal implications of LLMs, companies that prioritize responsible AI practices can also differentiate themselves. This could involve implementing robust measures to ensure data privacy and security, developing mechanisms to detect and mitigate bias in the models, and being transparent about the capabilities and limitations of the models. Companies that demonstrate a commitment to ethical AI will not only build trust with users but also be better prepared to navigate regulatory developments in the field. One could argue that Apple’s dominance is predicated on this very fact.
Though foundation models provide a step-function in natural language understanding and generation, new disruptors who focus on composability, data quality, and end-to-end automation will create long-term value at the app layer.
At NGP, we are interested to hear from early-stage companies building on top of foundation models to attack large enterprise pain points. If what you’re building fits within our core thesis of convergence or have any questions or feedback related to the post, feel free to reach out via LinkedIn or email to eric@ngpcap.com.
Blog image via Unsplash