Listen now
The broader definition of AI creates confusion, especially for those that may not be closely following the technology. Recent highly visible advancements driven by application of machine learning are sometimes misinterpreted and extrapolated to imply that we are at the threshold of imminent advance towards AGI, and all that it would entail for the societal order.
There is possibly a continuum of progress between IA and AGI technologies. I posit in this article that the rapid advances we are seeing represent an acceleration of IA technologies driven by machine learning. However, moving towards the original premise of AI — and AGI — would require significant additional technical breakthroughs beyond recent advances. IA technologies help advance human potential by increasing worker productivity, alleviating mundane tasks, and enhancing convenience in our lives. What we are seeing today is an acceleration in machines’ abilities to perform tasks that they have already been better than humans at for decades. And over the next decade, we will see further rapid advances along these fronts driven by further penetration of machine learning technology across many industries and spheres of life.
The State of Artificial Intelligence
Download our research briefing to dive into private market trends, startups working on ‘general AI’ or human-like intelligence, and the most prominent industries using AI algorithms.
What’s old is new
Many AI and machine learning algorithms used today have been around for decades. Advanced robots, autonomous vehicles, and UAVs have been used by defense agencies for nearly half a century. The first virtual reality prototypes were developed in the 1960s. Yet, as of late 2016, not a day goes by when a main stream publication doesn’t pontificate on the upcoming societal impacts of AI. According to CB Insights data, funding for startups leveraging AI will reach $4.2B in 2016, up over 8x from just four years back.
What has changed?
There are many factors at work, but there is consensus that many recent developments, such as massive recent improvements in Google translate, Google DeepMind’s victory at the game of Go, the natural conversational interface of Amazon’s Alexa, and Tesla’s auto-pilot feature, have all been propelled by advances in machine learning, more specifically deep learning neural networks, a sub field of AI. The theory behind deep learning has existed for decades, but it started to see renewed focus and a significantly accelerated rate of progress starting around the year 2010. What we are seeing today are the beginnings of a snowball effect in the impact of deep learning across use cases and industries.
Exponentially greater availability of data, cloud economics at scale, sustained advances in hardware capabilities (including GPUs running machine learning workloads), omnipresent connectivity, and low power device capabilities, along with iterative improvements in algorithmic learning techniques have all played a part in making deep learning practicable and effective in many day-to-day situations. Deep learning, along with other related techniques in statistical analysis, predictive analytics, and natural language processing, are already beginning to get seamlessly embedded in our day-to-day life, and across the enterprise.
Machines and Humans
Machines have long been significantly better than the human brain at several types of tasks, especially those that relate to scale and speed of computation. Three academic economists (Ajay A. et al) in a recent paper and HBR article posit that the recent advances in machine learning can be classified as advances in machine “prediction”:
“What is happening under the hood is that the machine uses information from past images of apples to predict whether the current image contains an apple. Why use the word ‘predict’? Prediction uses information you have to generate information you do not have. Machine learning uses data collected from sensors, images, videos, typed notes, or anything else that can be represented in bits. This is information you have. It uses this information to fill in missing information, to recognize objects, and to predict what will happen next. This is information you do not have. In other words, machine learning is a prediction technology.”
Completing any major task involves several components – namely data gathering, prediction, judgement, and action. Humans still significantly outpace machines at judgement-oriented tasks (widely defined), and Ajay et al postulate that the value of these tasks will increase as cost of prediction goes down with machine learning.
While there are purpose-built machines that can demonstrate selected human-like soft skills, machine capabilities in those areas have not made nearly as much progress as they have in the “prediction” function, driven by deep learning, over the past few years. Here are some areas that humans excel at, and new technology breakthroughs may be required for machines to emulate:
- Learning to learn: Most recent spectacular results from use of machine learning have been generated by machines that observe how humans act in a variety of instantiations of the task at hand (or large sets of inputs and outputs to the problem at hand), and “learn” using the deep neural network approach. Such machines are designed deliberately by a team of humans for a specific task, carefully generating the requisite training data and fine tuning requisite learning algorithms over a period of time. Machine learning techniques today could require orders of magnitude more training data than a human might for performing a similar task, e.g. a toddler can recognize an elephant after seeing a picture of one a few times, but a machine requires a much larger (and possibly primed) data set. Humans are good at learning to learn – they can learn a new skill completely unrelated to their current skill set, can decide what to learn and find and gather data accordingly, can learn implicitly/subconsciously, can learn from a variety of instruction formats, and can ask relevant questions to enhance their learning. Machines today are only beginning to learn to learn.
- Common sense: Humans are good at exercising “common sense,” i.e. judgment in universal ways without thinking expansively or requiring large data sets. Machines are in their relative infancy in this field, in spite of rapid strides in Natural Language Processing using deep learning. Scientists working on common sense reasoning reckon that additional new advances are required for machines to exhibit common sense. We (or our kids) have all faced this issue while trying to chat with Alexa or Siri.
- Intuition and Zeroing in: The human brain is good at exercising intuition and zeroing in, i.e. finding a fact, idea, or course of action from a very large, complex, ambiguous set of options. There are ongoing academic efforts and headways on bringing intuition to machines, but machine intelligence is generally very early on this dimension.
- Creativity: While there are now machines which have generated works of music or art that are indistinguishable to lay persons from the work of masters, these have been largely based on learning the creativity patterns of those masters. True creativity would entail the ability to generate novel solutions to problems not previously seen or to create truly innovative works of art.
- Empathy: ability to understand emotions, value system, setting a vision, leadership, and other soft capabilities remain uniquely human skills.
- Versatility: The same person can perform reasonably at many tasks, e.g. pick up a box, drive a car to work, console a kid, and give a speech. Machines and robots are still purpose built for specific tasks.
IA and AI
Summarizing the above – while machines have made rapid strides at learning or “prediction” skills, they are in their early days of attempting to emulate truly “human” skills. We propose that we classify prediction, first-order machine learning, and human-in-the-loop automation capabilities as “IA” technologies. These technologies typically use the unique power of machines (ability to process huge data sets) to effectively augment human capabilities, and the final output of the system typically depends on humans that bring complementary skills, those that train them, and those that design them.
Given its original roots, as well as the potential for confusion with AGI, the term AI should be reserved for machines that additionally demonstrate aforementioned human capabilities of judgement, common sense, innate creativity, ability to learn to learn, and empathy. This may still be short of the omnipotent AGI, but full automation of complex work flows would require machines that have most of these skills.
With this lens, here is how one could classify some current and upcoming technologies expected to impact our daily lives and work:
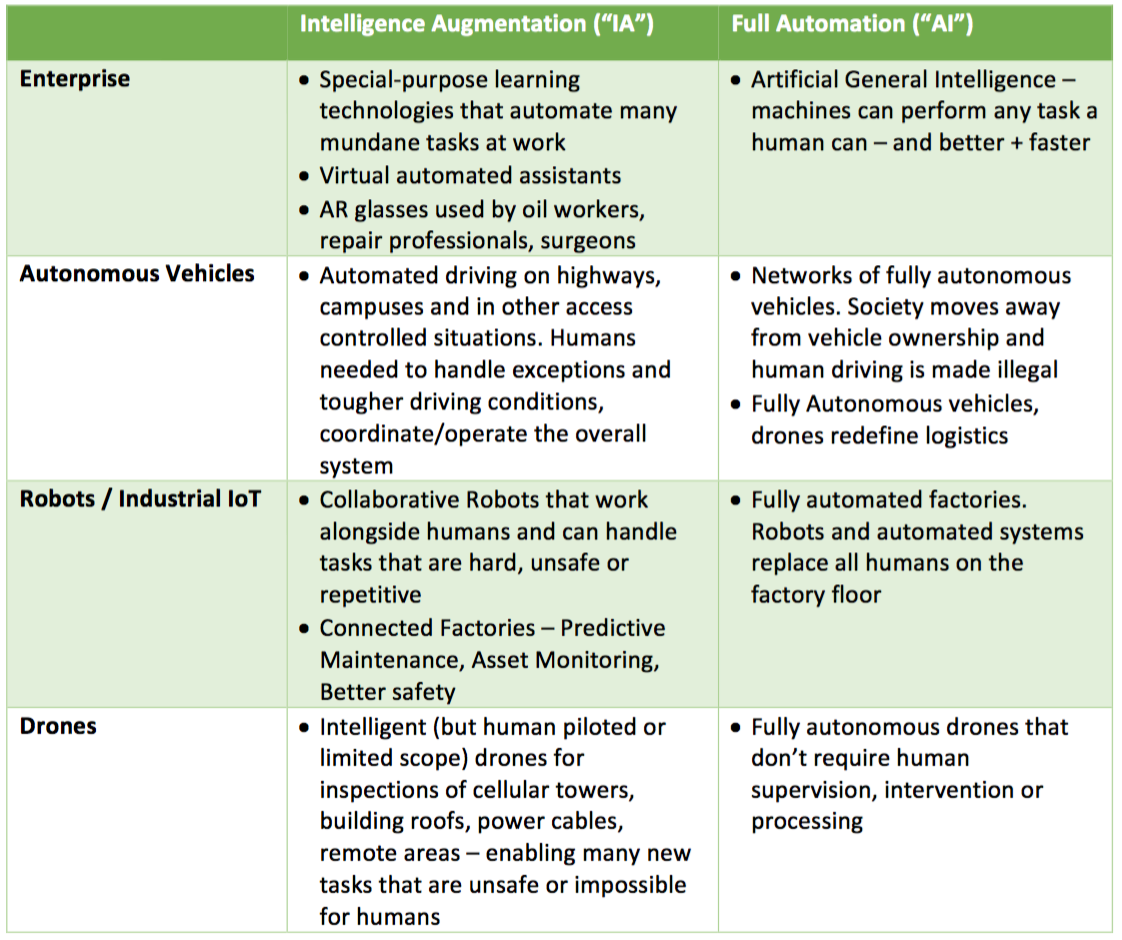
Impact of IA and AI
It is a well-known adage that we tend to overestimate the impact of technology in the short term, but underestimate it in the longer term. This is also known as “Amara’s Law,” and often represented by the illustration below.
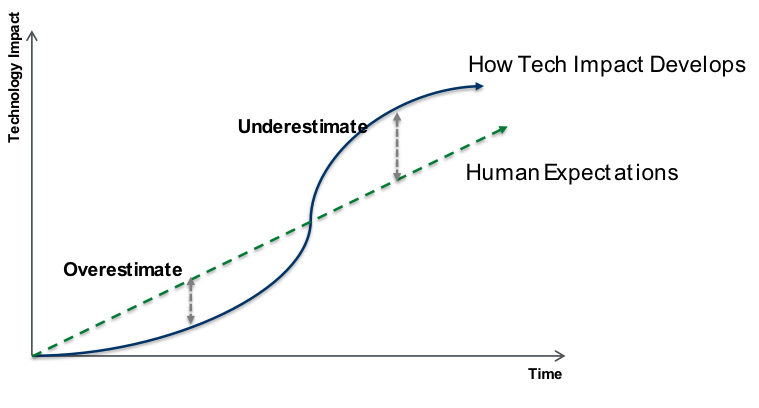
It is noteworthy that this curve does not have a scale on either axis. And at any point at the beginning of the curve, we don’t accurately know how far out that inflection point is. But it does illustrate an important tendency well — that the impact of new technology breakthroughs is initially slow to take off, then accelerates significantly as the technology develops and reaches mass market adoption, and eventually saturates. Human and market expectations tend to overlook this trend. This then feeds into the well-known market hype cycles where human expectations initially get well ahead of the immediate impact of the technology, thereby creating the milieu for the dip into the trough of disillusionment. As the tech impact then continues to expand and reaches broad scale, the technology reaches the plateau of productivity.
I believe that what we are seeing today in the form of significant developments and awareness around the term “AI” is really an acceleration of a prior “IA” curve, where deep learning using artificial neural networks (along with aforementioned drivers around hardware, data, cloud economics, connectivity, and other algorithmic advances) is driving us towards an inflection point on that curve. In many scenarios, deep learning provides an upgrade over prior “prediction” methods that used regression and other statistical tools, rules-based systems and/or hand-coded logic. Machine learning is providing significant acceleration here by improving model accuracy, increasing ability to process data, and being more adaptive to inputs.
In light of aforementioned limitations that machine intelligence still has, I think that the curve for full automation technologies should be seen as an entirely new one. And I believe we are still in very early days of the full automation curve.
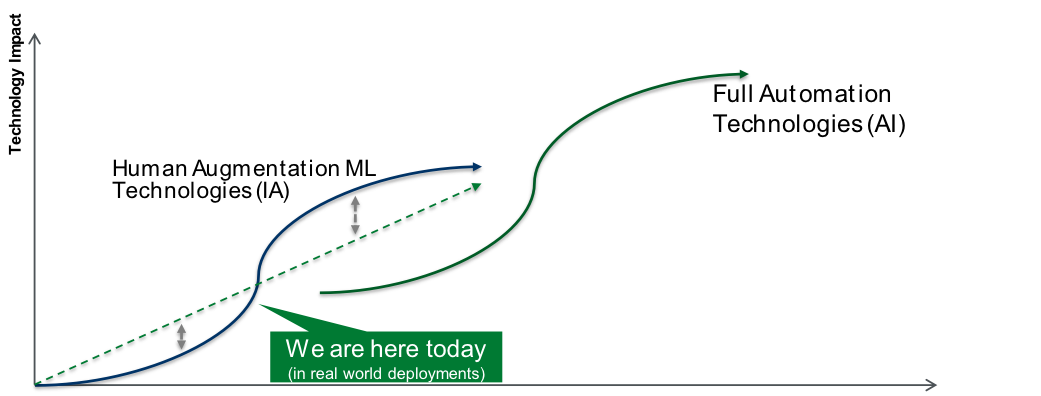
There have been multiple prior hype cycles and inaccurate predictions around AI and singularity. Many AI pioneers during its early days in the 1950s believed that machines with full human-like capabilities would exist within ten or twenty years. The reason we didn’t get there was not that computing power did not advance fast enough, but that scientific breakthroughs on multiple new dimensions were required for that to happen. And the timing of such fundamental breakthroughs is hard to predict and coordinate. According to Stephen Hawking, as of 2015, “There’s no consensus among AI researchers about how long it will take to build human-level AI and beyond.”
We are likely close to a local maxima in the hype around “AI.” However, IA (as defined above) presents a very large investing opportunity with a 5- to 10-year horizon. Humans and machines at the current point in time complement each other, excelling at different types of skills. This will enable humans to focus on skills that they uniquely possess and enjoy exercising, while machines handle most of the mundane tasks that do not require human skills of judgement, creativity, and empathy. A lot has been written about the changes that IA technologies would entail to the nature of work and jobs, and change is not going to be easy for all. This Wired article summarized it well: “You’ll be paid in the future based on how well you work with robots.”
Where are the immediate innovation and investment opportunities?
I believe that the impact of IA (human-enhancing automation using deep learning and other machine learning techniques) would be bigger in the medium term than most think, while full automation is further away than some recent reporting might indicate.
The point of this post is not to speculate on whether AGI is 10 years away or 100, or whether or not it is a threat to society. The point I am making is that if you are investing in or starting a company or project today with up to a 5- to 10-year horizon to achieving mass scale, there is significant immediate value in IA or human augmentation technologies propelled by machine learning, and that these present a lower resistance path to commercial and societal success.
As always, for B2B models, we are looking for offerings that: address a clear existing pain point, can demonstrate strong ROI, fit neatly into existing work flows, are able to align interests with the buyer, user, and facilitator in the enterprise, and are a pain killer rather than vitamin. In this space, I would bet on human-in-the loop (IA) technologies, which help increase overall productivity, optimize costs, personalize solutions, or help business offer new products to their customers.
Machine learning technologies are being applied to many aspects across various vertical industries. This is a topic for an entire investment thesis (or at least a separate post of its own), but here is a short list of sectors where companies are leveraging advances in machine learning to augment human capabilities, enhance productivity or optimize use of resources:
- Enterprise – Robotic assistants that automate mundane, repetitive tasks are coming up across a variety of functions, and will penetrate deeper across the enterprise fabric over the next decade. Wearables using augmented reality will help smoothly accomplish tasks that are unsafe or expensive today.
- Manufacturing – Collaborative, intelligent robots that can safely work alongside humans and can handle tasks that are hard, unsafe, or repetitive will help increase productivity.
- Transportation and Logistics – While there is a fierce ongoing public race amongst various technology companies and OEMs to drive towards fully autonomous vehicles, there is significant nearer term opportunity from reducing driver work load in mundane driving situations such as highways, reducing error rates and accidents in human-driven vehicles, and improving traffic flow and fuel efficiency. Over a period of time, the fully autonomous ecosystem promises to change the fabric of urban life, and will create many concomitant opportunities.
- Healthcare – Machine learning technologies that can help medical professionals with more accurate, personalized diagnosis based on wider data sets.
- Agriculture – A variety of farming robots, crop optimization techniques, automated irrigation systems, and pest warning systems will help increase agricultural productivity at high rates.
The 100 year Study on Artificial Intelligence being conducted by a preeminent academic panel recently published a balanced report with a good overview of opportunities and progress in machine learning technologies across many of these sectors. CB Insights has a compendium of how deep learning is being applied by innovative startups across a broad set of industries.
Time for a change in terminology
As machine intelligence moves well beyond its erstwhile realm within the technology industry, and into various “traditional” industries, it is beginning to touch numerous people who are not versed with the technical terminology around AI techniques.
We would be well-served by being more nuanced in the use of the term “AI.” To avoid confusion, risk of adverse sentiment and regulation, and to better recognize the upcoming era of abundance, we should switch to using a term such as “IA” to describe recent advances achieved from using machine learning techniques. I believe that IA better describes the human-machine symbiosis that the impact of the current breed of technologies depend on. I am not suggesting something unprecedented. As machines become more capable, mental facilities once thought to require intelligence are typically removed from the definition of AI. E.g., Optical Character Recognition was once considered an AI technology, but having become a routine technology, is not generally considered so now.
Let’s use the term AI solely to describe full automation technologies, which, as we have argued, are not necessarily a direct extrapolation of the curve we are on today. And in the meanwhile, let’s capitalize on opportunities arising out of rapid recent strides in IA technologies.