In the rapidly evolving landscape of cloud infrastructure, NGP has consistently championed the concept of Edge Cloud as the next-generation of infrastructure. In our journey to advance this paradigm, we have identified a crucial obstacle that hinders its widespread adoption: the "second app problem". In this article I explore the role of foundation models for edge use cases.
One key insight I have had about edge cloud as a space is that it suffers from a second app problem – where buyers are focused on a single use case and want to procure a solution for just that use case. Not having a second app is often a limiting factor as budgets are not allocated from a strategic perspective. This makes it challenging for truly horizontal infrastructure companies to take advantage of strategic budget allocation.
Regardless, doing AI at the edge has a potential to unlock high-impact use cases in verticals such as industrial, manufacturing, and logistics thus defining a new era of digital industries. From this standpoint, I have been investigating edge AI platforms, particularly the application of foundation models to edge AI. I asked industry practitioners and startup founders who are building edge AI solutions a key question: Can foundation models be applied to edge AI problems? The following are three key insights I gathered based on the conversations:
Insights #1: For foundation models to be truly served at scale, hybrid edge architecture needs to be adopted mainstream.
The best performing foundation models are extremely large with billions of parameters. The current thinking around foundation models has been that they require massive amounts of training data and are so resource intensive that they cannot be ported to an edge device for inferencing. But there is on going research to reduce the storage and compute overhead for model training and inferencing at the edge. Meta’s LlaMa models, for example, are already optimized for running on PCs, while Google’s announcement of its next generation of PaLM 2 models talked about a version for the edge called Gecko. Furthermore, research indicates that smaller models trained for longer can give good, if not better results, further increasing the possibility of not just inferencing but also training at the edge.
Qualcomm has been a huge proponent of hybrid AI architecture and on device AI and we think they are on point. Hybrid AI architecture could either be a) purely device centric or b) device sensing hybrid AI. In the case of pure device centric architecture, the model dimensions, inputs and outputs are kept such that it can function entirely on the device and the contact to cloud is initiated only if absolutely necessary or for federation purposes. In the case of device sensing hybrid AI, the device acts as the eyes and ears and the act of inferencing are split between the device and the cloud. Both these architectures will play a crucial role in truly serving foundation models at scale. They have benefits such as bringing down inferencing cost, improving energy efficiency, maintaining privacy while improving personalization etc.
Insights #2: Multi-modal models are going to be the defacto norm at the edge
When people first hear the word foundation model, they are likely to associate it with GPT or large language models (LLMs). The scale and research on language models has been unprecedented. But multi-modal models are a growing subsect within deep learning that deal with the fusion and analysis of data from multiple modalities such as text, images, video, audio, and sensor data. Multi-modal deep learning combines the strengths of different modalities to create a more complex representation of data, leading to better performance on various machine learning tasks.
Given the complexity of the use cases of applying AI at the edge, multi-modality will be driving force for the adoption of edge AI. Improvements in building and operationalizing multi-modal models will be crucial for making AI at the edge a reality. Entirely new workflows will emerge for computer vision model development. Even in multi-modal model use cases, language will continue to be the primary input modalities given the accessible nature of language as a modality. For multi-modal models, we are yet to solve problems around enterprise readiness (such as hallucinations) – a problem that is an active area of research for language.
Insight #3: A new light weight container is needed for inferencing at the edge
This is an interesting white space we identified for inferencing to happen at the edge. Given the heterogeneity of devices at the edge, what we need is a container that truly decouples hardware from the software a.k.a docker for edge. Startups such as Fermyon, a leader in the WebAssembly (WASM) space have been trying to claim this white space. Although WebAssembly as a technology is very interesting and could be used to create light weight edge applications for performance critical tasks, I am yet to come across enterprise adoption of the same. For now, light weight container for edge AI is a white space, and companies solving for container related problems such as orchestration, management, cost optimization, etc., are yet to emerge.
The tech stack for using foundation models for edge AI use cases is still in the works but below is the first cut of what the stack would look like.
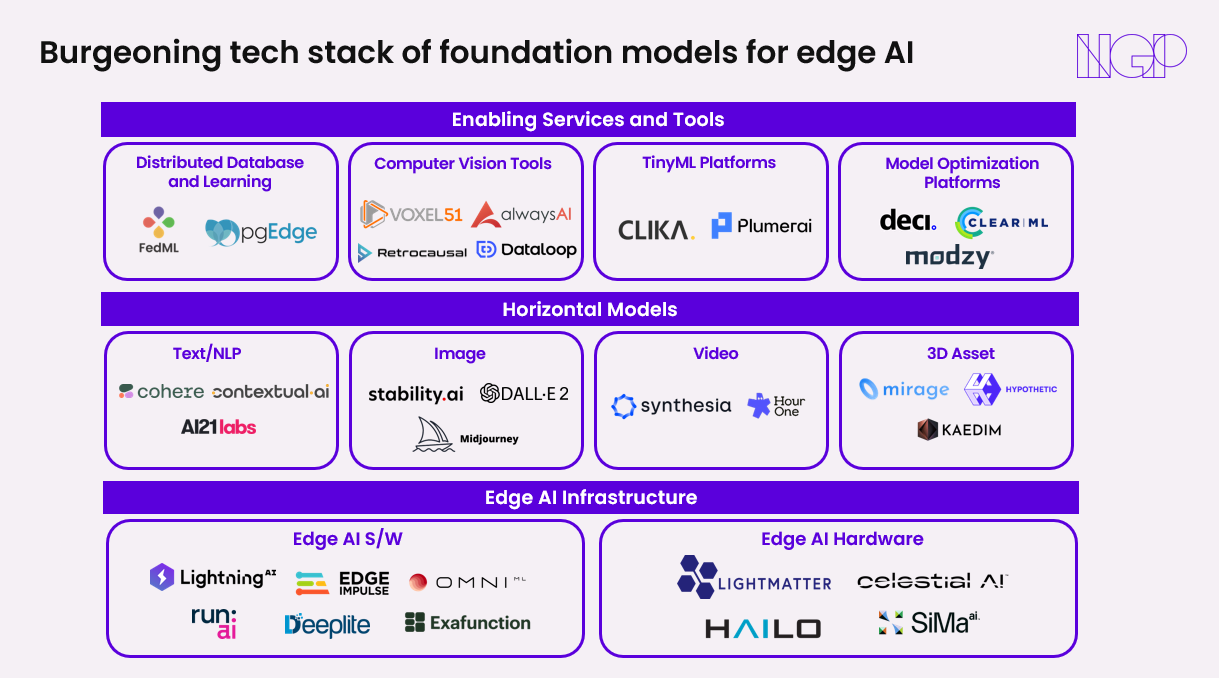
We at NGP have been bullish about the edge AI space. With portfolio companies such as Xnor (acquired by APPL), Scandit, etc., we are constantly looking to increase our footprint in this space. If you are building a company in this space, please feel free to reach out to us. We’d love to get to know you!